
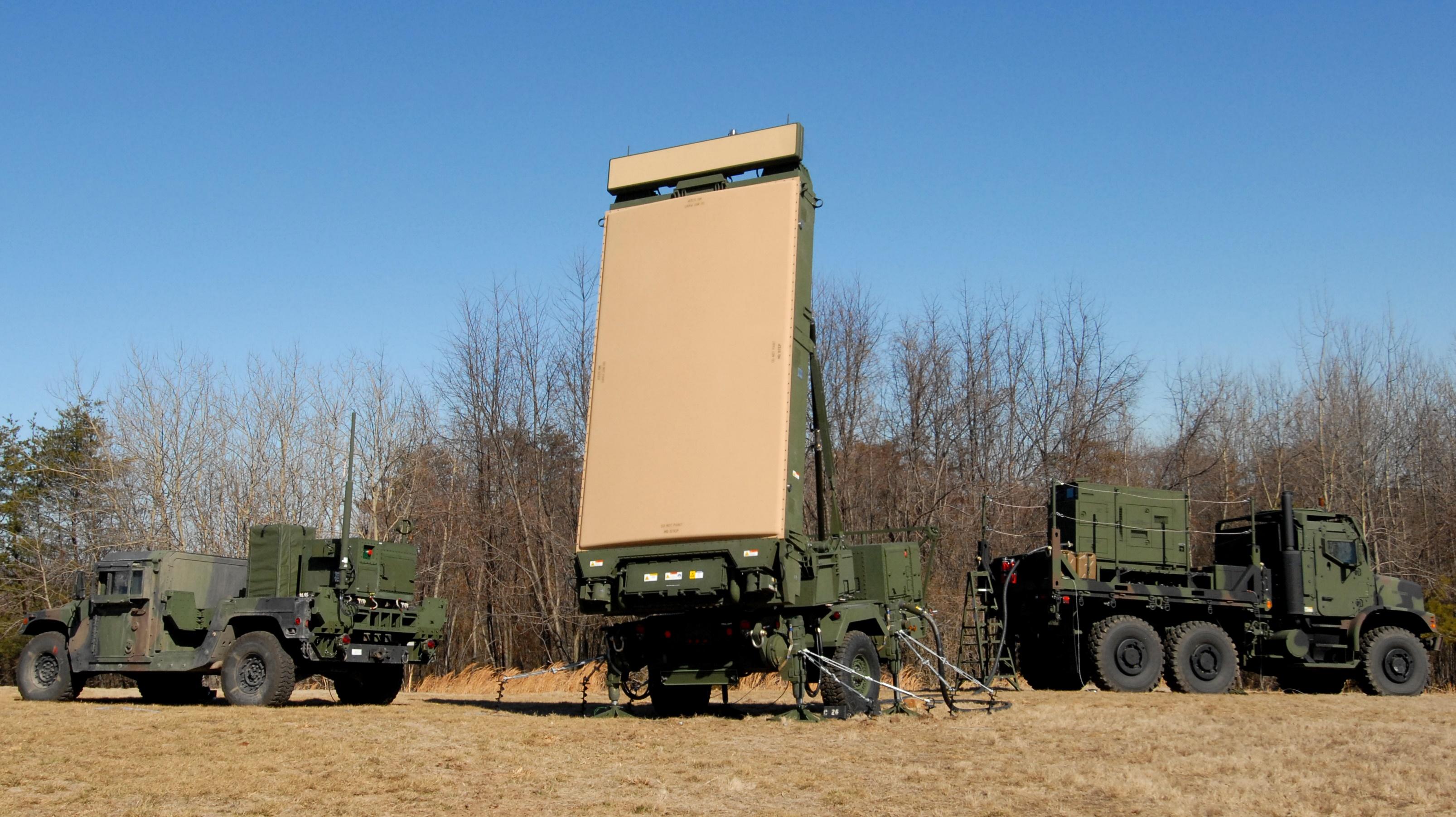
#Network radar 2.3.1 how to
How to effectively recognize active deception jamming is a challenge of modern radar technology. Similarly, by changing the network input, the original signal is used to replace the echo signal, which improves the accuracy of the jamming recognition in the case of a low JNR.Īctive deception jamming is one of the common means to jam radar signals. Using a CNN to classify the time–frequency image has realized the recognition of a variety of common deceptive jamming techniques. This method fuses three short-time Fourier transform time–frequency graphs disturbed by three consecutive pulse periods into a new graph as the input of the convolutional neural network (CNN). This paper studies the input of jamming recognition networks and proposes an improved intelligent identification method for chirp radar deceptive jamming. The existing neural network-based jamming identification methods still follow the pattern of signal modulation-type identification, so there are fewer types of jamming that can be identified, and the identification accuracy is low in the case of low jamming-to-noise ratios (JNR). Traditional deceptive jamming recognition methods need to extract complex features and artificially set classification thresholds, which is inefficient. Radar active deceptive jamming based on digital radio frequency memory (DRFM) has a high coherence with the target echo, which confuses the information of the target echo and achieves the effect of hiding the real target. Simulation results show that the proposed method can classify and recognize radar emitter signals of different modulation types in parallel under low SNRs.The perception of jamming types is very important for protecting our radar in complex electromagnetic environments. Finally, time-frequency image is recognized and classified through the model, thus completing the automatic classification and recognition of the time-domain aliasing signal. Secondly, the multi-label classification and recognition model for multi-modulation radar emitter time-domain aliasing signals is established, and learning the characteristics of radar signal time-frequency distribution image dataset to achieve the purpose of training model. The time-frequency distribution image is then denoised using a deep normalized convolutional neural network (DNCNN). First, we perform time-frequency analysis on the received signal to extract the normalized time-frequency image through the short-time Fourier transform (STFT). This method can quickly perform parallel classification and recognition of multi-modulation radar time-domain aliasing signals under low SNRs. This paper proposes a multi-label classification and recognition method for multiple radar-emitter modulation types based on a residual network. In low signal-to-noise ratio (SNR) environments, the traditional radar emitter recognition (RER) method struggles to recognize multiple radar emitter signals in parallel.
